Passing the GenAI tipping point: The role of infrastructure in ongoing innovation
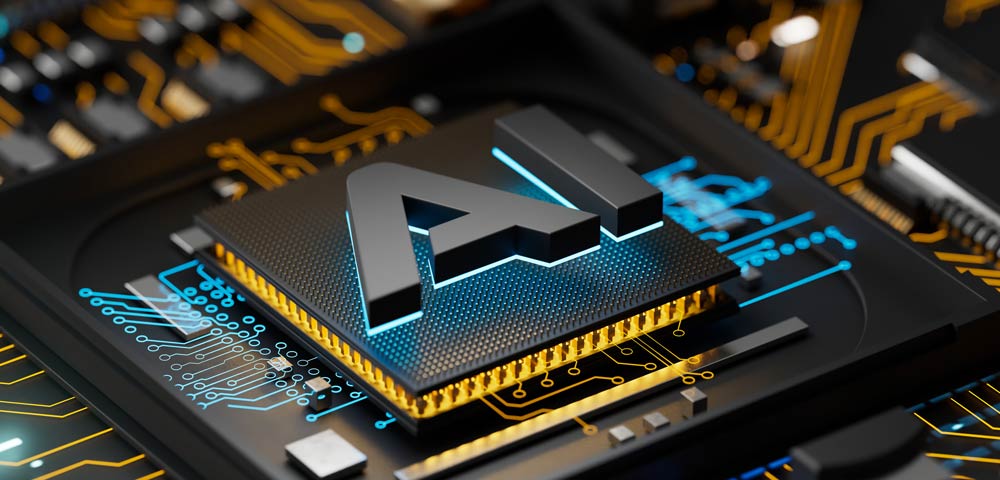
Computer scientists have been making advances in AI technology for decades. But a convergence of factors is driving its massive momentum in the current moment.
In recent years, public interest in AI, and generative AI in particular, has exploded. With more and more enterprises adopting this transformative technology, the scale of opportunity for companies providing the vital infrastructure undergirding GenAI tools is also on the rise.
At the 19th annual KeyBanc Capital Markets Emerging Technology Summit in March, moderator Eric Heath, Senior Equity Research Analyst covering security, Data and AI, convened a panel of thought leaders in this dynamic field: Ed Anuff, Chief Product Officer, DataStax; Jeff Boudier, Head of Product + Growth, Hugging Face; and Yan-David Erlich, Chief Operating Officer, Weights & Biases. During the session, the group discussed the current momentum of GenAI, the role infrastructure plays in its evolution, and what the future of the field could look like.
A pivotal moment for GenAI
Computer scientists have been studying and making advances in AI technology for decades. But a convergence of factors is driving its massive momentum in the current moment. First, as Erlich pointed out, the launch of ChatGPT, self-driving cars, and other AI tools for public consumption has made the technology more visible and tangibly functional than ever before. And while AI models have existed for many years, the volume of computing power and available data on which to train those models has only recently reached a threshold that makes these innovative applications possible. The recent introduction of transformer AI models, a deep learning architecture developed by Google, has upped the GenAI game. Finally, the proliferation of pre-trained, adaptable generative AI models across various platforms and communities has facilitated a “Cambrian explosion” of the quantity and diversity of models in existence.
Open vs. closed foundation models
AI tools are built on foundation models, which can be open or closed. Foundation models are trained on a broad, generalized data set and can perform tasks such as processing language and generating text and images. Closed models are developed privately, often by organizations that want to use and maintain proprietary control of the models for competitive reasons. In contrast, open AI models’ creators make them publicly available. An open model’s architecture, parameters, and in some cases the completed, trained models are shared for other researchers and developers to study and adapt.
Closed models tend to leverage application programming interfaces (APIs), which makes them easier to “plug and play” than open models, which require developers to build their own tech stacks. Closed models also tend to be highly capable in terms of performance. However, Boudier of Hugging Face — an open platform for AI builders — noted that the performance of open models is catching up and, in some cases, even surpassing that of closed models.
Whether to adopt an open- or closed-model approach depends on the goals and needs of each organization. Cost and performance also play a role: “The open models right now are generating significantly better, consistent performance with much more predictable cost,” said Anuff.
Looking ahead, Erlich envisioned that GenAI models will continue to evolve for a variety of use cases, with different models excelling at different tasks. For enterprises looking to leverage the technology, the most effective approach may be building some proprietary models while adapting capabilities of existing models via transfer learning and fine-tuning.
The role of databases
Because generative AI models depend on massive troves of data for training purposes, databases are a crucial element of their infrastructure. The development of vector databases, which encode a user’s request as a vector that allows an AI model to look up relevant data within a database, has been an important element of GenAI’s evolution. However, not every database is a vector database, and models may need to interface with multiple different kinds of databases.
For Erlich, there’s value to building specialized databases for different use cases. A database that stores contacts on a smartphone is rightfully not going to look or function like a database that stores readings from an internet of things (IoT) sensor in a manufacturing laboratory. Determining what kind of database is appropriate depends on each system’s consumption of resources. As Anuff described, when it comes to translating what the user is looking for into a query the AI model can answer, a vector database is the simplest process, but not the only one. He pointed to developments in text to SQL that can generate useful queries into Snowflake and other systems.
GenAI goes mainstream
More companies are now rolling out frameworks, tools, and databases for building GenAI applications, and that infrastructure is becoming increasingly useful to the 25 million software developers around the world. According to Anuff, this will likely result in an “explosion in application development” over the next 18 months. “The ability to put GenAI to work in mainstream applications is at a point where it’s never been before, and it’s rapidly accelerating,” he added. Boudier agreed, and noted that the Hugging Face platform now hosts over a million AI models, data sets and applications. “It’s past the ML research niche that we were in four years ago, and has broken through to developers and companies.”
Erlich reminded the audience that the public may not yet see the positive impact that GenAI is making in many enterprises. “It’s not some chatbot they’re using to talk to their customers, but their individual employees are more productive, happier at work, doing more creative tasks, and actually producing better output as a result of collaborating with machine learning systems.”
From supporting drug discovery in the pharmaceutical industry, to facilitating the emergence of self-driving vehicles, to improving risk models in banking, the world has only begun to scratch the surface when it comes to applications of generative AI — and the infrastructure driving this technology will play a critical role in its ongoing evolution.
Reach out
To your KeyBanc Capital Markets investment banker, or relationship manager to discuss this article, and the potential impact on the growth of your business.
Visit KeyBanc Capital Markets for more information.
About the Emerging Technology Summit
The 19th annual Emerging Technology Summit, held in San Francisco, California, brought together executives and founders from the top emerging private and public companies, thought leaders, and investors to network, discuss trends and outlooks, and generate ideas for 2024 and beyond. The forum was packed with high-quality, personalized, and intimate meetings among companies and investors, complemented by impactful keynotes, thematic panels, and engaging fireside chats and presentations. If you would like more information about attending this conference in the future, contact our Corporate Access team.
This article has been prepared and circulated for general information only and presents the authors’ views of general market and economic conditions and specific industries and/or sectors. This report is not intended to and does not provide a recommendation with respect to any security.
KeyBanc Capital Markets is a trade name under which corporate and investment banking products and services of KeyCorp® and its subsidiaries, KeyBanc Capital Markets Inc., Member FINRA/SIPC, and KeyBank National Association (“KeyBank N.A.”), are marketed. Securities products and services are offered by KeyBanc Capital Markets Inc. and its licensed securities representatives, who may also be employees of KeyBank N.A. Banking products and services are offered by KeyBank N.A.
Securities products and services: Not FDIC Insured • No Bank Guarantee • May Lose Value
Please read our complete KeyBanc Capital Markets disclosure statement.